;)
Integrating Machine Learning and Imaging Technologies in Anti-infective Drug Discovery
In contrast to conventional approaches, ArrePath identifies compounds with new and under-exploited mechanisms of action at the very outset of the drug discovery campaign, optimizing for efficacy, safety, and other critical attributes once the mechanism and target engagement are defined.
Our ‘mechanism-first’ and knowledge-led approach enables significant efficiencies in the drug discovery and optimization process, as well as an early and critical understanding of a compound’s potential addressable target product profile and clinical utility.

Phenotypic profiling and bacterial autopsies
Decoding complex biology and bacterial autopsies, utilizing machine learning-enabled imaging and microscopy
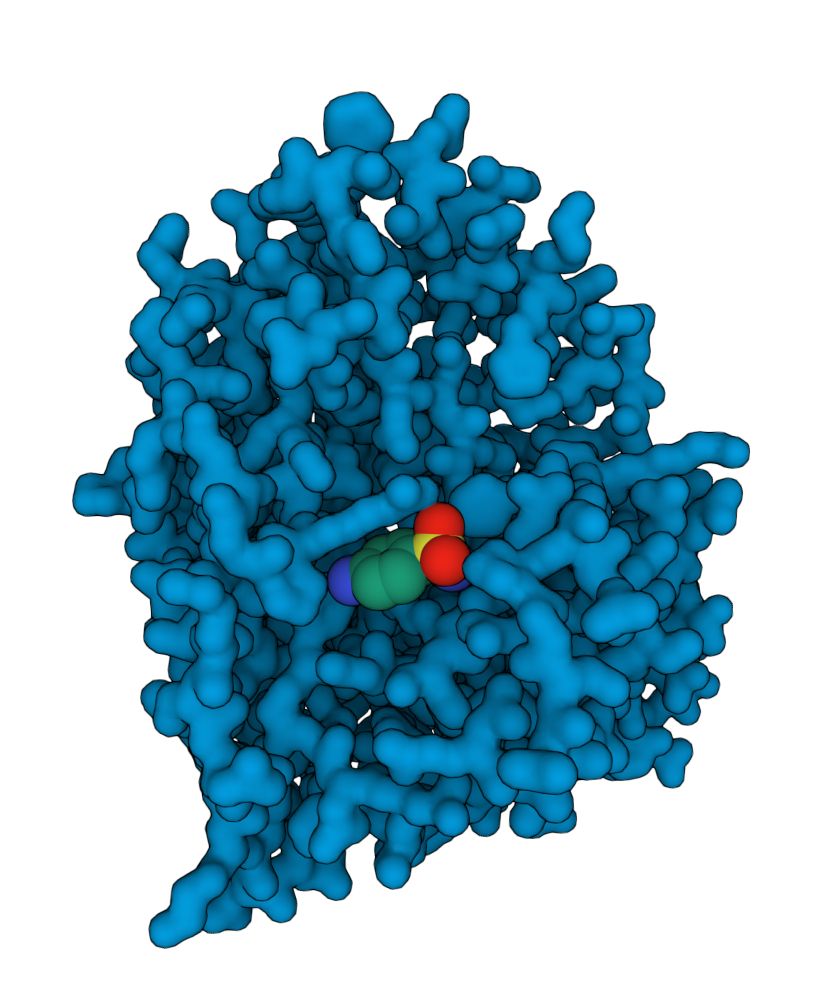
MOA analysis and confirmation
Rapid confirmation of mechanism of action / target engagement. Deep analysis of multidimensional phenotypic signatures combined with proteomics and genomics technologies
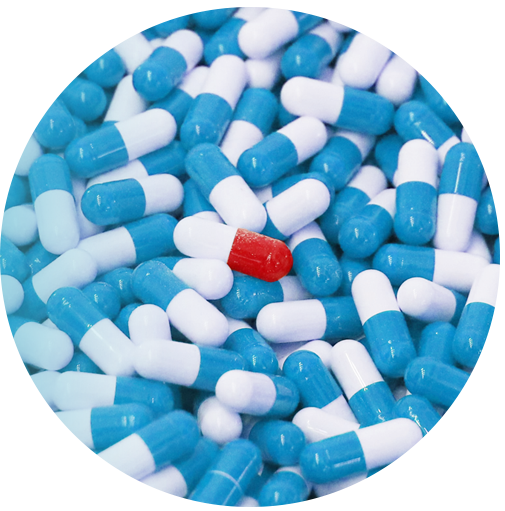
AI / ML enabled drug design
AI / ML enabled multi-parameter optimization to rapidly optimize hits to leads, delivering candidates
Instead of using standard growth inhibition assays, ArrePath integrates multidimensional phenotypic screening, proteomics, and genomics-based technologies with machine learning-powered analysis of the resulting rich and highly detailed data ‘landscape’. This entire process enables rapid identification of the mechanism of action of hits, which are then prioritized for further optimization. The sensitivity and data-rich nature of ArrePath’s approach also allows identification of weaker hits that affect biological pathways that would have been previously deprioritized using ‘traditional’ technologies, yielding potential tractable starting points for new classes of antibiotics.
ArrePath utilizes its imaging-based platform during the hit to lead and lead optimization process to assist in the efficient development of structure activity relationships in cell-based systems. We also integrate machine learning and artificial intelligence to guide complex multiparameter optimization that addresses the multiple challenges in antibacterial drug discovery. Specifically, this approach allows the company to optimize for potency, spectrum of activity, cell wall penetration and efflux avoidance, cytotoxicity, and DMPK parameters, to move an early compound to preclinical development candidate status, addressing specific Target Product Profiles.
Our Platform at a Glance
The behavior of cells when exposed to an external influence such as a chemical entity is exquisitely linked to the mechanism of action of that compound. We use proprietary imaging and machine learning workflows to extract a phenotypic signature from this resulting behavior. This process can be used like a ‘bacterial autopsy’ to identify new antibiotics; by understanding how the cell died, we can understand what it died of.
We obtain phenotypic signatures for cells treated with a large number of marketed drugs and other compounds to provide a multidimensional map of features. This allows us to quickly identify the mechanism of action of new compounds identified from initial screening campaigns by comparing their ‘autopsies’ to our detailed ‘global’ map.
Technology and Workflow
ArrePath has developed innovative workflows to determine the specific mechanism of action of lead compounds, after initial bacterial cytological profiling (BCP) through the application of imaging and machine learning technologies. The application of proteomics and genomics technologies then allows us to confirm target engagement and define what is responsible for antimicrobial activity.
Novel Targets
Bacterial ‘autopsies’ enable the rapid identification of mechanism of action, target ‘novelty’ and hence whether it is ‘under-exploited’ and of potential clinical utility.
Target Focused
We can also focus on specific targets of interest by searching for compounds that work through mechanisms linked to high potential of clinical utility or demonstrated clinical utility.
Rapid & Efficient
Once a confirmed hit is identified in an initial screen, the process of determining and confirming its MoA is highly efficient.
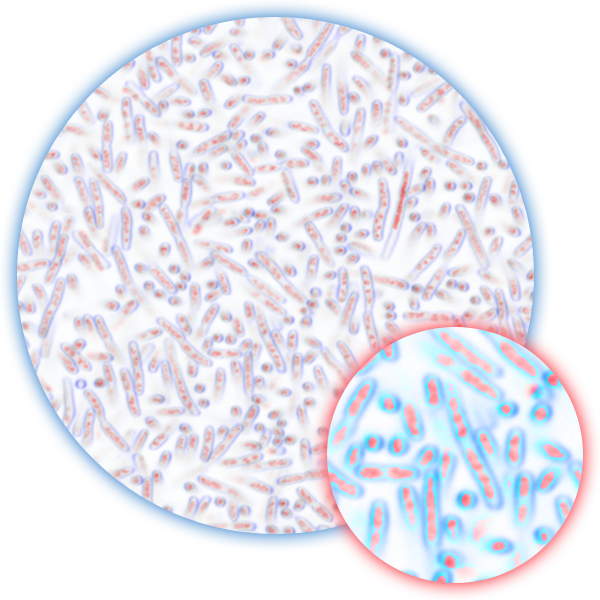
Our Platform’s Proof-of-Concept
Proof-of-concept of the ArrePath platform has been demonstrated in studies published in Cell by our scientific founder, Professor Zemer Gitai, Ph. D., who has pioneered this approach. The process identified a compound with a novel, dual mechanism of action that is active against both gram-negative and gram-positive bacteria with no detectable resistance.
The research led to the discovery of the specific elements of the dual mechanism, showed they are synergistic, and that their synergy depends on distinct moieties contained within a single molecule. The initial lead compound led to a series called the ‘Irresistins’, and the platform has identified additional compounds with novel mechanisms, with potential as starting points for optimization and future development. ArrePath has exclusive rights from Princeton University to intellectual property related to the platform technology and novel compounds generated through its application.